Dr. Ravi Parikh Launches the Human Algorithm Collaboration Laboratory (HACLab)
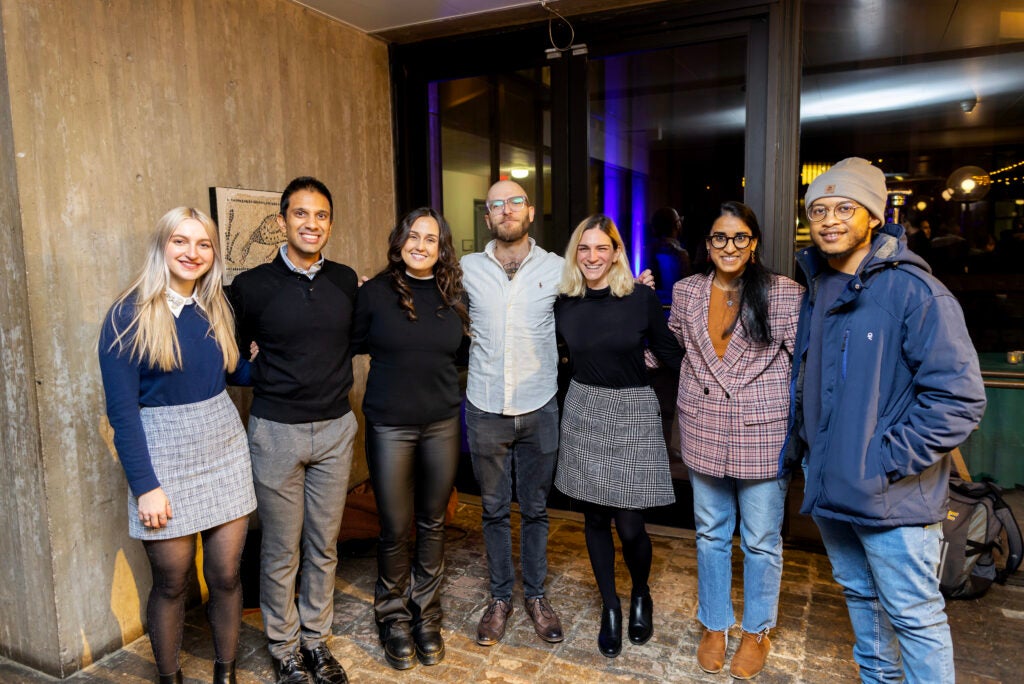
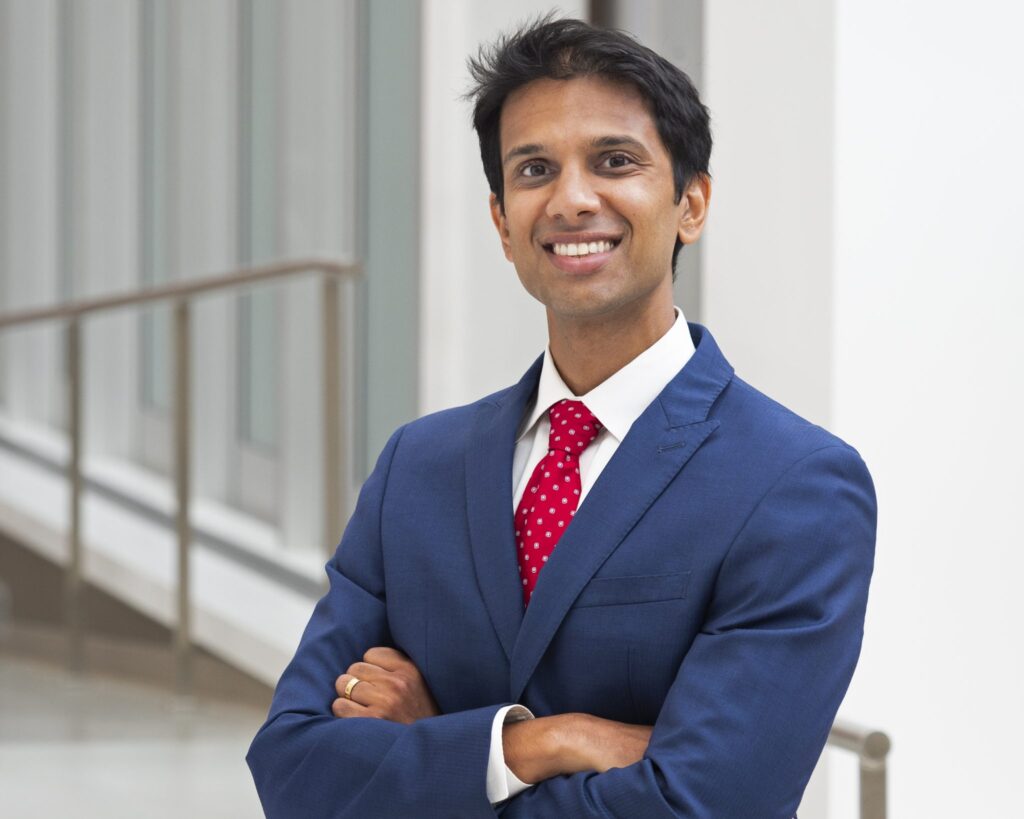
Ravi Parikh, MD, MPP, FACP, Assistant Professor of Medical Ethics and Health Policy and Medicine at the University of Pennsylvania and a CHIBE-affiliated faculty member, just launched the Human Algorithm Collaboration Laboratory (HACLab). CHIBE spoke with Dr. Parikh and his team for a Q&A about this new lab, which implements and scales artificial intelligence (AI) and machine learning.
What is the mission of the HACLab?
Our mission is to train, validate, implement, and evaluate interventions based on AI, machine learning, and predictive algorithms to improve patient health and reduce health inequities. We work with clinicians, data scientists, behavioral economists, human factors scientists, biostatisticians, and policymakers to answer key questions in patient care through targeted clinical trials and observational studies.
In my opinion, three things make the HACLab different from a typical AI/machine learning lab.
First, we are pretty much entirely focused on implementing and scaling AI and machine learning. That means that most of our projects are not foundational in pushing the boundaries of machine learning and AI methodologies. And we also do not focus on building models that may be accurate but are too complex to ever be scalable in health care settings. Rather, we design practical AI and machine learning models that respond to discrete needs from clinicians, patients, and end-users. That involves sitting down with end-users to get a sense of what should and should not go into an algorithm, how should algorithm outputs be presented, and how sensitive or specific would the end-user want the algorithm to be. This input is necessary before we start building algorithms. We then partner with organizations to implement these models in practice.
Second, we run trials that investigate how AI can augment clinicians and patients, rather than replacing them. That means that we very rarely run a straight-up algorithm vs. human comparison; instead, we usually test an AI-augmented solution against an existing standard of care (either clinicians’ judgement or algorithm alone). We think AI augmenting clinician or patient judgement is the more realistic comparison for clinical care, and this type of comparison allows us to see which settings humans predict or diagnose better than machines and vice versa.
Third, we are more than just algorithm-builders! For example, we have a robust program producing policy-focused work on how AI should be regulated, reimbursed, and subject to liability. None of these models are fully baked, which means that we have a chance to frequently interact with government and industry to shape the next generation of AI policy in healthcare.
How is your team using behavioral economics with this Lab’s work?
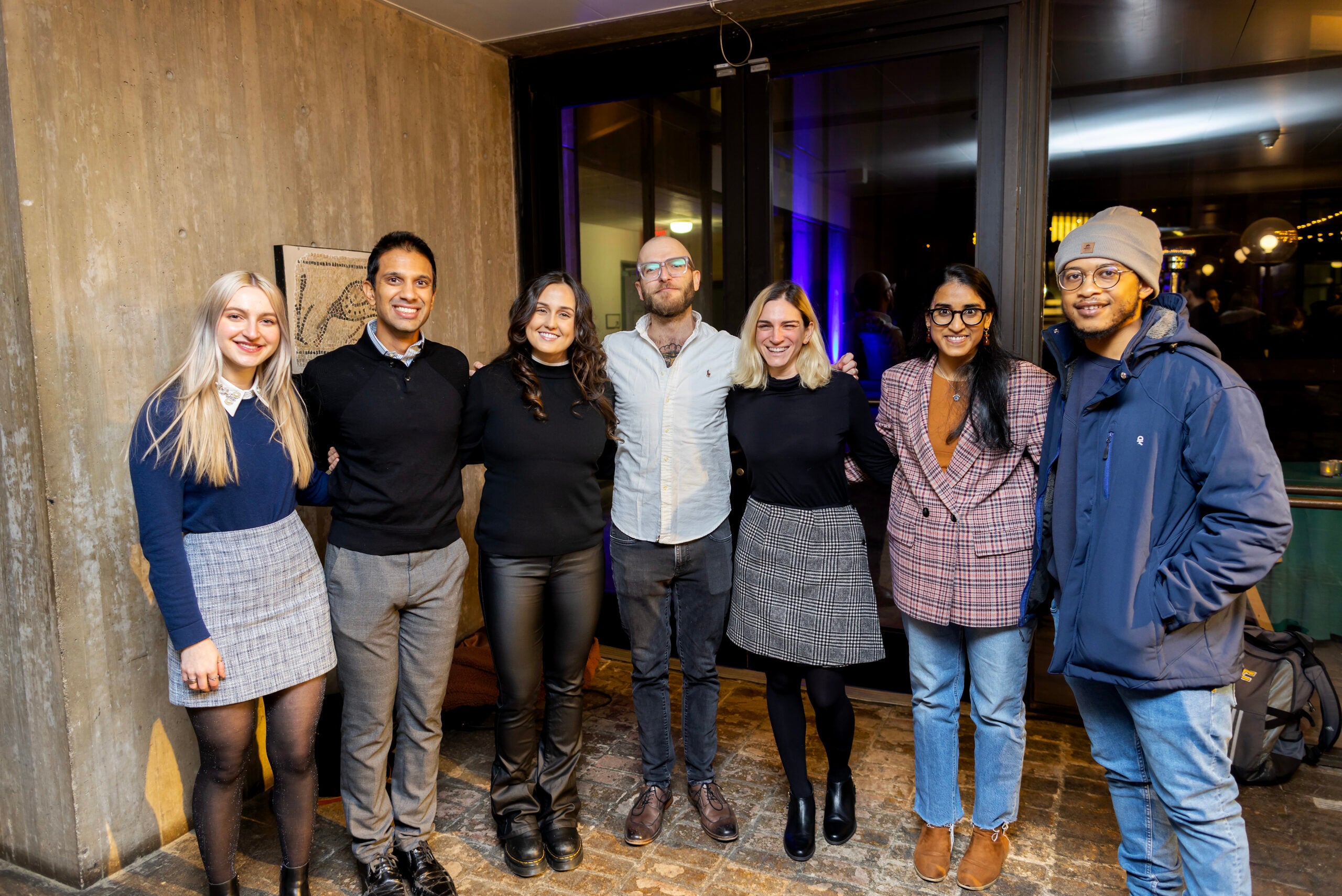
Behavioral economic principles are necessary to solve the “last mile” problem with AI and machine learning: How can we design and implement AI-based interventions to improve clinical care? First, we can’t just assume clinicians or patients will automatically listen to what the machine is predicting. Even the most perfect algorithm may not convince a hardheaded physician to act upon a prediction. We’ve used behavioral economic strategies – including defaults or accountable justification – to increase the likelihood that the machine prediction prompts the desired action from a clinician.
Second, even if patients or doctors agree with an AI’s prediction or diagnosis, there are ways that we can use behavioral economic principles as part of a “kitchen-sink” intervention. For example, we designed a trial using a machine learning-based intervention to increase rates of serious illness conversations among patients at risk of dying from cancer. We didn’t only rely on the machine predictions to convince physicians to have more conversations. We also used peer comparisons and performance reports to help prime physicians to the behavior. In that way, we can augment the impact that the machine learning algorithm can have.
What’s the importance of human touch in AI?
First, humans are really good at predicting certain things and can see information that machines cannot see. Say you have a machine learning algorithm that is predicting someone’s risk of dying in the hospital. Well, the machine can only generally see and analyze things in the computer – electronic medical records or images, for example. Clinicians, however, can gain a huge amount of predictive information just from looking at the patient in front of them; that’s information that the machine will never have. So, when we design models, rather than pitting “human vs. machine,” we are experimenting with ways to incorporate human prognostic and diagnostic information as input features to make machine learning algorithms better.
Second, we need a human touch to make the outputs of AI more actionable. Say that you are in clinic, and you receive a cold notification on a screen that says, “Your patient has a 65% chance of benefiting from this treatment.” That might be an accurate estimate, but it doesn’t do anything to ensure the prediction is acted upon. We can use input from humans to structure predictive outputs in ways that prompt the right decisions and actions from physicians. That’s the real definition of human-algorithm collaboration.
Give us a sense of the range of projects that this Lab is working on right now and in the future.
When it comes to AI and machine learning, we have two flavors of work: Foundational and Applied.
In Foundational work, we build and train models and study how such algorithms could impact clinical care. We are currently focusing on statistical methods to detect and mitigate bias in AI (with Amol Navathe and Kristin Linn), to detect “drift” in the performance of algorithms before that drift can actually harm patients (with Jinbo Chen and Likhitha Kolla), and to improve the “explainability” of AI so that doctors can trust predictions that AI generates (with Qi Long and Mayur Naik). Zeke Emanuel and I, along with collaborators at Michigan, MIT, and Manganese Solutions, just received a grant to use machine learning to improve risk-adjustment algorithms among tens of millions of Medicare beneficiaries.
In our Applied work, we are applying fixed models to influence clinical care decisions. One example is the project I mentioned above, where we used machine learning to increase rates of serious illness conversations (with Christopher Manz, Justin Bekelman, and Mitesh Patel). That has spawned a new line of work (with Justin Bekelman and Sam Takvorian) where we work with community-based partners to develop algorithm-based solutions to increase palliative care utilization and improve end-of-life care. In a similar project with Carmen Guerra, we are using machine learning algorithms to identify patients at high-risk of colorectal cancer to target navigation programs that can help patients get their colonoscopies. Finally, we are using a technique called natural language processing that can scour millions of lines of unstructured text in the electronic health record to identify a patient’s likelihood of being eligible for a clinical trial, which may help improve the efficiency of clinical research coordinators’ jobs and improve equity in clinical trial enrollment.
How could a government entity or business work with the HACLab?
By reaching out to us at our email (haclab@pennmedicine.upenn.edu) or contacting us via our website or social media. Many of our collaborations are with industry partners who want to test their algorithm in a rigorous trial or use machine learning to improve the efficiency of an operation. We are interested in having conversations with industry and government entities in addition to help shape regulation, bias, liability, and reimbursement considerations around AI and machine learning in healthcare.
What are some upcoming events planned for HACLab?
We have weekly lab meetings and quarterly get-togethers as a lab. We’d love more ideas of how our lab can collaborate with other groups in formal and informal settings!
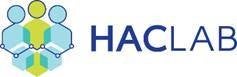